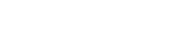
Non-adversarial generative models are relatively easy to train and have less mode collapse than adversarial models. However, they are not very accurate in approximating the target distribution in latent space because they don't have a discriminator. To this end, we develop a novel divide-and-conquer model called Tessellated Wasserstein Auto-Encoders (TWAE) which has less statistical error in approximating the target distribution. TWAE tessellates the support of the target distribution into a given number of regions using the centroidal Voronoi tessellation (CVT) technique and designs data batches according to the tessellation instead of random shuffling for accurate computation of discrepancy. Theoretically, we demonstrate that the error in estimating the discrepancy decreases as the number of samples n and the regions m of the tessellation increase at rates of \mathcal {O}(\frac{1}{\sqrt{n}}) and \mathcal {O}(\frac{1}{\sqrt{m}}) , respectively. TWAE is very flexible to different non-adversarial metrics and can significantly enhance their generative performance in terms of Fréchet inception distance (FID) compared to existing ones. Furthermore, numerical results demonstrate that TWAE is competitive to the adversarial model and shows powerful generative ability.
Publication:
IEEE Transactions on Pattern Analysis and Machine Intelligence, vol. 46, no. 2, pp. 780-792, Feb. 2024
http://dx.doi.org/10.1109/TPAMI.2023.3325282
Author:
Kuo Gai
NCMIS, CEMS, RCSDS, Academy of Mathematics and Systems Science, Chinese Academy of Sciences, Beijing, China
School of Mathematics Sciences, University of Chinese Academy of Sciences, Beijing, China
Shihua Zhang
NCMIS, CEMS, RCSDS, Academy of Mathematics and Systems Science, Chinese Academy of Sciences, Beijing, China
School of Mathematics Sciences, University of Chinese Academy of Sciences, Beijing, China
Email: zsh@amss.ac.cn
附件下载: